How to analyse qualitative data
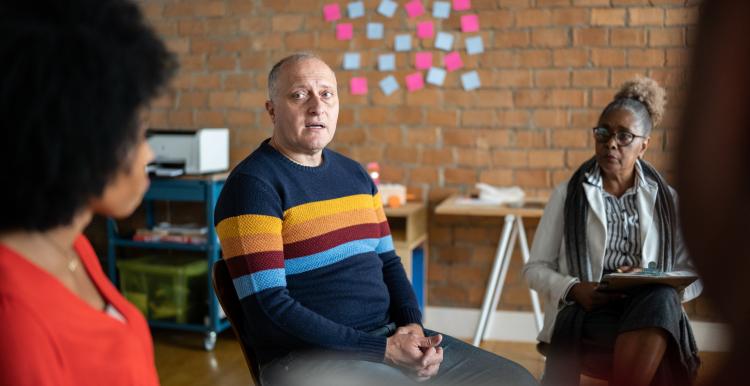
Contents
- About this resource
- What is qualitative data, and why is it important to analyse?
- Before you start your analysis
- Five steps to help analyse your data
- Data saturation
- Top tips for reporting qualitative data
- Further reading
About this resource
Qualitative data is information that captures people's views, emotions, thoughts, and attitudes. Analysing qualitative data allows you to draw meaningful insights and discover patterns and relationships in your evidence.
This guide will help you recognise the steps you need to follow when analysing qualitative data so that you can improve your data analysis skills and produce impactful reports. There are top tips for reporting your analysis as well.
What is qualitative data, and why is it important to analyse?
When you analyse non-numeric information, such as interview transcripts, notes, audio/visual recordings, or even images, you are analysing qualitative data.
Qualitative data analysis generates nuanced information and helps you understand the "why" and the "how". It enables you to comprehend experiences, phenomena, and context. At Healthwatch, you often collect qualitative data as free-text during one-to-one interviews, focus group discussions, feedback and signposting activities, or even via surveys.
Qualitative data analysis takes longer than quantitative data analysis because the researcher needs to read the free text before drawing on the insights. When analysing qualitative data, it's important to put oneself in another person's shoes and see things from their perspective.
An example of qualitative data analysis
Many elective care procedures were cancelled or rescheduled at the pandemic's start. You are interested in finding out how this impacted people waiting for the treatment.
You conducted telephone interviews with 15 such people who had their appointments cancelled by the hospital.
You find out that the cancellations had impacted people differently on analysing the interview transcripts. It helps you draw themes from your data, such as the impact on their physical health, mental health, income and finances, and people's social life and relationships.
You also compare your findings against the demographics of your participants. You note that participants in their 70s had indicated a loss of mobility and became housebound when the hospital cancelled their procedures.
It helps you draw on the relationship between loss of mobility and the feeling of isolation, especially among the elderly.
Note that you cannot assign a numerical figure to your findings. It can only help to discover and understand human experience.
Before you start your analysis
- Organise your data – have your complete data set in one location, systematically arranged and easy to look at. You can use dedicated qualitative analysis software to help you keep your data organised, such as MAXQDA, NVivo or Quirkos.
- Mark any vital information that will help you with the analysis, such as participant demographics or location.
- Think about what you want to find out but be open-minded about unexpected discoveries.
- Stop analysing for a theme when you reach data saturation to avoid wasting time and resources. Scroll towards the end of this guide to learn more about data saturation.
- Be aware of researcher bias and take steps to minimise it.
How to minimise researcher bias in qualitative data analysis
The personal beliefs of a researcher can influence their analysis and the results. While eliminating bias may not be entirely possible, you can minimise it by triangulating your data.
Triangulation means using two or more methods to verify your findings and results. You can triangulate in three ways:
- Methodology triangulation – collecting data using different methods, e.g., conducting in-depth individual interviews and a focus group discussion.
- Investigator triangulation – having more than one researcher to analyse the data. If there is consistency in their findings, you can be confident about the analysis.
- Data triangulation – using multiple data sources, e.g., comparing your findings with similar research done by another local Healthwatch or another organisation.
Remember, triangulation can help you cross-validate your findings and capture different dimensions of the same phenomenon.
Five steps to help analyse your data
1. Decide on your analytical approach
Before you do any analysis, think about how you will approach it. You can create a coding framework or use a thematic network analysis approach. You can also use a combination of the two.
Coding framework: Creating a coding framework means you decide the themes/topics you would be looking for in your data before the analysis. The codes help you create structured pre-determined labels which you can attach to the text in your data. You can use the codes to generate themes or link multiple codes to understand the relationship between the themes.
You could analyse using a coding framework when you undertake a piece of primary research, which has clear aims and objectives set right from the start. It means that you already know the themes you are interested in even before starting the analysis.
Thematic network analysis: On the other hand, the thematic network analysis approach is exploratory. You develop codes and themes as you read your data. It starts without preconceived ideas and allows the data to help build a picture and an overall story.
You could analyse using the thematic network analysis approach when looking at your feedback and signposting information or data gathered at a community engagement event. In these cases, you will explore the data without preconceived themes to determine what is happening in the local health and social care landscape.
Examples of uses these approaches
Coding framework: You are doing a project on digital exclusion and have done interviews with people who struggle to access digital healthcare. To find out the relationship between income and digital exclusion, you can create an "Affordability" code. You can also develop sub-codes to explore more in-depth information about affordability. The sub-codes can be:
- Income types and levels
- Type of telephone handset
- Type of telephone/internet contracts
- Cost of broadband/internet connection
- Access to IT hardware, e.g., laptops, webcams, smartphones
Thematic network analysis: In the above example, as you read through your data, you discover other implications of limited income. For example, you might find out that some people depend on their family to buy telephones or computers and even pay internet bills. Therefore, they may lack the freedom of choice about accessing remote care and could become digitally excluded if the person they depend on cannot pay. The thematic network analysis approach helps you establish a new relationship between digital exclusion and dependencies on others.
2. Review and explore your data
The next step is to read through your data, perhaps several times, to understand what it contains.
If you are unsure about something, you could ask the participant or another person who knows about it.
You should aim to try and understand the true feelings of your participants based on what they have said. Focus on their stories and what they mean for them.
Pay attention to the words used, particularly the intensity of the word. For example, "I felt uneasy about asking the doctor again, so I left" can be different from "I felt embarrassed to ask the doctor again, so I left". The latter is more intense than the former.
People with limited English may not express themselves adequately. It would be best if you tried to understand what they say rather than focus on the choice of words they have used. For example, if someone says, "I don't feel comfortable because I feel not good, I feel for example I have a problem plus my stress because of the language barrier". You can infer that they cannot express their concerns due to language barriers. And they feel stressed when they are unable to communicate.
3. Code your data and build overarching themes
Organise the words or phrases into codes. Then build a theme or multiple themes with the codes, giving deeper meaning to your data.
Example of building an overarching theme
In the previous example, you looked at participant interviews to discover why people are digitally excluded. As you read the transcripts, you code your data as follows:
- Technology skills
- Interest in technology
- Privacy/trust issues
- Sensory/dexterity impairments
- Fluency in English
The codes help you develop a theme – people are digitally excluded for various reasons. The theme enables you to establish the finding that several factors, including lack of interest or skill, language barriers, and disabilities, can make a person digitally excluded. It can impact their interest to engage with online healthcare services.
4. Validate your analysis and the findings
As discussed, it is good practice to validate your analysis and findings to reduce the possibility of bias. If only one investigator is undertaking the research, they must follow a consistent coding and analysis method. If more than one staff is involved, make sure they follow a systematic approach. A coding framework with a clear rationale for every code is an excellent way to ensure your researchers are consistent.
Once you have coded and analysed the data, ask a team member to do the same for a small sample of the data. You can compare their finding with yours to check for any differences. If there are differences, it doesn't necessarily mean one is correct and the other is wrong. You may have discovered another finding in the process.
You can also ask for feedback from your research participants to check if your findings justify their opinions and sentiments.
5. Explain your findings
The essential last step of the process is to explain the meaning of what you have found. Reread your themes and findings and try to form a summary. Tying your themes together should help you get a better idea of the overall results and help you develop an accurate narrative of the data.
Relate the findings to the people who took part in your study. It is also the best time to look for differences in people's opinions from different demographic groups.
The importance of demographic data analysis in qualitative research
As the COVID-19 vaccination started rolling out in January 2021, we analysed feedback from people about their views and experiences of the vaccine. Although most people were optimistic about the vaccine, some weren't.
We then looked at the evidence by people's ethnicity and found that vaccine hesitancy was relatively higher in people from specific ethnic minority communities. They remained uncertain whether taking the vaccine was right for them. To understand what was lacking from the current roll-out strategy and help address concerns, we commissioned research to look at groups with lower vaccine take-ups.
The above example highlights why demographic analysis is essential. It helped us identify a specific issue – higher rates of vaccine hesitancy in particular communities – and undertake further in-depth conversations with people from these communities. It helped us understand the reasons behind their hesitation and take a step towards addressing health inequalities.
Data saturation
When undertaking qualitative data analysis, it is helpful to think about data saturation. Data saturation is the amount of qualitative data which is adequate to develop an accurate and robust understanding of the answers to your research question. Once you reach data saturation, any new information will not add additional value to your research. It would be best to stop collecting more data or analysing it to avoid wasting time and resources.
Reaching data saturation in qualitative research depends on a few factors, such as what is your research question, who are your participants, your sampling and data collection method, and how rich is the information in your data.
If you are undertaking a qualitative research project at Healthwatch, typically involving 12 to 15 in-depth interview participants would help you reach data saturation. You can carry out a few more interviews or observations to be sure.
An example of data saturation
You carry out research to explore the impact of remote GP appointments. You interviewed 14 people who have used their GP services during the COVID-19 lockdown. When you analyse the data, you discover that almost all had indicated that they found it difficult to book an appointment over the phone. It helps you establish that the most common area of dissatisfaction with remote care was the appointment booking process over the phone. You do not need to conduct more interviews and analyse more data to establish that GP surgeries must improve their telephone appointment booking process.
Top tips for reporting qualitative data
- Include a summary at the start of your report highlighting your key findings.
- Use your findings to tell a story – people often engage with stories better than they do with numbers and statistics.
- Demonstrate your findings with quotes from your research participants. It helps your readers connect with the experiences of another human being.
- Do not use multiple quotes to support the same finding – one to two quotes per argument is plenty.
- Clean the quotes so they are readable. For example, correct spelling or grammatical errors, insert [missing words] in brackets, shorten long quotes with ellipses, and use quotation marks to make your quotes distinct. It is also helpful to write quotes in a larger font and/or different style. You can also use identifiers with your quotes to make them more relatable. For example:
“I don’t want to use them [computers] and I don’t feel I should have to be forced to do this. I am a hands-on bloke and expect a hands-on approach…I think you should have a relationship with your doctor – I prefer to see the same doctor as I like that personal touch.” White British male, 58 years old.
- You can also include visual elements, such as images or infographics to make your qualitative findings more engaging. Try to choose images that are unique and specific to your work.
Further reading
Read more about how to turn your findings into a report.
Find out how to analyse quantitative data in your research.